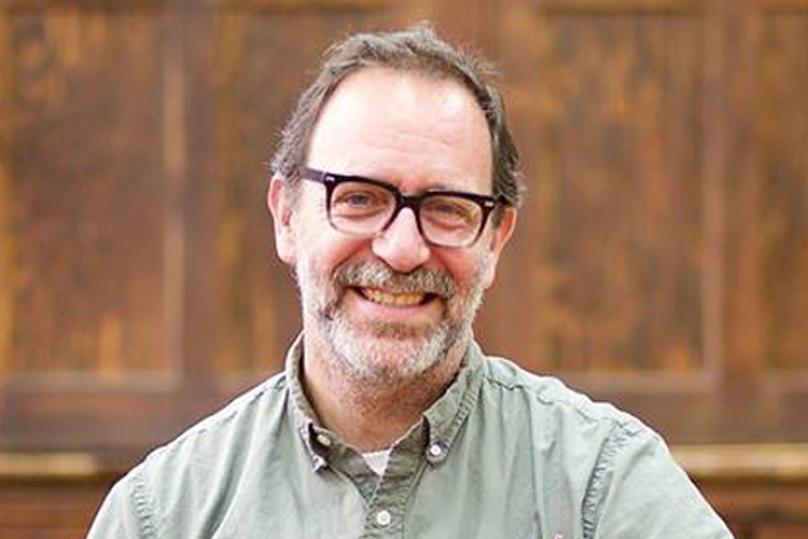
MƒA knows that many teachers have an interest in learning about current and foundational education research in their content area – and who better to provide this insight than a practicing Master Teacher.
New to the MƒA Teacher Voices blog is this focus on important and practical academic research articles in mathematics.
Doug Shuman, two-time MƒA Master Teacher and mathematics teacher at Brooklyn Technical High School, will summarize key mathematics pedagogy research articles over the school year. We hope, over time, this will grow to serve as a valuable resource for both our teacher community and the greater education community.
Article of Focus:
Burger, William F. and Shaughnessy, J. Michael, Characterizing the van Hiele Levels of Development in Geometry, Journal for Research in Mathematics Education, Vol. 17, No. 1 (January, 1986), pp. 31- 48. NCTM.
Summary:
We’ve all seen geometry students’ thinking transform over the course of the year. Often, however, students struggle with some idea or concept that seems obvious to us. It can be frustrating to help students because they can’t see a relationship or generalize its properties even when it feels like we’re giving away the answer. This phenomenon was extensively explored forty years ago by the husband-wife pair of Dutch education researchers Dina van Hiele-Geldof and Pierre van Hiele. They developed a theory involving levels of thinking that they asserted students must pass through sequentially. These levels have come to be called the van Hiele Levels of geometric reasoning:
- Level 0 (Visualization). The student reasons about basic geometric concepts by means of visual considerations of the concept as a whole without explicit regard to properties of its components.
- Level 1 (Analysis). The student reasons about geometric concepts by means of an informal analysis of component parts and attributes.
- Level 2 (Abstraction). The student logically orders the properties of concepts, forms abstract definitions, and can distinguish between the necessity and sufficiency of a set of properties in determining a concept.
- Level 3 (Deduction). The student reasons formally within the context of a mathematical system, complete with undefined terms, an underlying logical system, definitions, and theorems.
- Level 4 (Rigor). The student can compare systems based on different axioms and can study various geometries in the absence of concrete models.
The van Hieles’ research sparked interest but drew criticism over the difficulty in assessing appropriate student levels. In their 1986 paper, “Characterizing the van Hiele Levels of Development in Geometry,” William Burger and J. Michael Shaughnessy attempted to validate the van Hiele levels and develop a set of indicators that would allow teachers to assign students to levels.
They did this by creating a set of tasks and interview questions given to 45 Oregon students from Kindergarten through college. Researchers observed, interviewed and recorded students as they worked individually on tasks. The tasks were of different levels of cognitive demand, given the variety of the students’ ages. The tasks involved drawing shapes, identifying and defining shapes, sorting shapes, and engaging in both informal and formal reasoning about geometric shapes.
When the analysis was completed the researchers were left with a catalog of indicators that can be used to assign a van Hiele level. For example, “inability to conceive of an infinite variety of types of shapes,” is an indicator of Level 0 (Visualization). Burger and Shaughnessy found that the van Hiele levels are generally descriptive of student performance but that they are less hierarchical and more dynamic, allowing students to be at different levels for different tasks, alternating between two levels on a given task as their thinking develops, and bi-directional when regression occurs over time without practice.
Using these indicators does not require a commitment to yet another assessment system. It is overkill to assign a van Hiele level to every student on every task. Rather, a good read of the indicators coupled with thoughtful reflection on what difficulties your students may have with a specific topic may lead you to better focus your pedagogy. For example, on a given topic one might assume that students are able to explicitly reference definitions (Level 2, Abstraction), when, in fact, they are focused more on assessing and comparing various properties (Level 1 Analysis). A thoughtful activity might begin with their ability to list various properties and then develop minimum sufficient conditions for classifying the shape.
A striking feature of the list of indicators is how language-based the differences between the levels are. Most of the distinctions come down to differentiating between relevant and irrelevant properties, and then developing abstract classifications that lead to verifying conjecture through formal deduction. Students can work through these phases by discussing concepts in a structured setting. Providing opportunities for conversational verbalization and explication is a great way for students to solidify understanding and transition to the next level.
Berger and Shaughnessy make the observation that the levels may be less discrete than fluid, especially between Level 1 (Analysis) and Level 2 (Abstraction), a fact particularly relevant to high school geometry teachers. Most geometry texts and curricula merge these two levels into one, with more focus on analysis than abstraction, assuming that once a basic level of explication and classification has been achieved, students are able to move quickly to Level 3 (Deduction). More time spent on abstraction, (eg. Always, Sometimes, Never exercises) is an investment that pays for itself.
My final thought on this paper is a word of warning: Since you and your students are on different levels, many will not be able to understand your line of thinking, even if you explain it to them. Eager to advance, some students will resort to memorization, a sure sign that your pedagogy is misaligned with their van Hiele level. Abiding this will only lead to mutual frustration.